Coefficient Of Variation And Machine Learning Applications (Intelligent Signal Processing And Data Analysis)

In the realm of data analysis and signal processing, the coefficient of variation (CV) stands as a pivotal metric for assessing the relative variability within a dataset. This quantitative measure provides insights into the dispersion or spread of data points around the mean, offering valuable information for a wide range of applications in machine learning (ML) and intelligent signal processing.
Definition of Coefficient of Variation
The coefficient of variation is defined as the ratio of the standard deviation (σ) to the mean (μ) of a dataset:
CV = σ / μ
4 out of 5
Language | : | English |
File size | : | 22688 KB |
Text-to-Speech | : | Enabled |
Enhanced typesetting | : | Enabled |
Print length | : | 148 pages |
Screen Reader | : | Supported |
It is expressed as a percentage, allowing for easy interpretation and comparison across different datasets. A lower CV indicates less variability, while a higher CV signifies greater variability.
Applications in Machine Learning and Intelligent Signal Processing
The CV finds extensive use in various ML and intelligent signal processing applications, including:
1. Data Normalization and Standardization:CV can serve as a valuable tool for normalizing and standardizing data. By dividing the standard deviation by the mean, CV brings data points to a common scale, facilitating meaningful comparisons and analysis.
2. Feature Selection:In feature selection, CV can help identify features that contribute significantly to the variability of a dataset. Features with high CV indicate greater potential for discrimination and can be prioritized for further analysis.
3. Anomaly Detection:CV can be leveraged for anomaly detection by identifying data points that deviate significantly from the average variability. This information can assist in flagging outliers or unusual patterns that may require further investigation.
4. Signal Denoising:In intelligent signal processing, CV can be employed to estimate the noise level within a signal. By comparing the CV of the original signal to the CV of a filtered or denoised signal, the effectiveness of noise removal techniques can be evaluated.
5. Model Evaluation:CV plays a crucial role in model evaluation by providing insights into the model's performance. A lower CV for model predictions indicates better stability and reliability, while a higher CV suggests higher uncertainty or potential overfitting.
Interpretations of Coefficient of Variation
The interpretation of CV depends on its specific application:
1. Relative Variability:CV provides a measure of relative variability, indicating the extent to which data points are dispersed around the mean. It is particularly useful for comparing the variability of different datasets or features.
2. Robustness of Statistics:CV can assess the sensitivity of statistical metrics to outliers. A dataset with a high CV is more likely to be influenced by extreme values, whereas a dataset with a low CV exhibits more robustness.
3. Process Monitoring:In industrial settings, CV can be used for process monitoring to track the stability and consistency of a process. A sudden increase in CV may indicate process disturbances or equipment malfunctions.
Limitations of Coefficient of Variation
While CV is a valuable metric, it also has certain limitations:
1. Sensitivity to Outliers:CV can be heavily influenced by outliers, which can skew the measure of variability. It is recommended to use robust statistical methods that are less sensitive to extreme values.
2. Non-Interpretability of Values:CV values themselves do not convey any specific meaning or units. Therefore, it is important to interpret CV in the context of the specific application and data distribution.
3. Not Suitable for Categorical Data:CV is not directly applicable to categorical data, as it assumes a continuous distribution. Alternative measures, such as the variance ratio or entropy, may be more appropriate for categorical data.
Best Practices for Using Coefficient of Variation
To ensure effective use of CV, the following best practices are recommended:
1. Consider Data Distribution:Understand the underlying distribution of the data before applying CV. Normal or near-normal distributions yield reliable CV estimates, while non-normal distributions may require logarithmic transformation or robust statistical methods.
2. Use Robust Statistical Methods:Whenever possible, use robust statistical methods (e.g., median and interquartile range) to reduce the impact of outliers on CV calculations.
3. Compare Relative Variability:When comparing CV values, it is important to consider the relative variability between different datasets or features. Absolute CV values may not be directly interpretable across different applications.
Alternative Measures of Variability
In addition to CV, several alternative measures of variability can provide valuable insights in specific scenarios:
1. Interquartile Range:The interquartile range (IQR) represents the difference between the 75th and 25th percentiles of a dataset. It is less sensitive to outliers than standard deviation and provides a measure of variability for non-normal distributions.
2. Mean Absolute Deviation:Mean absolute deviation (MAD) measures the average absolute distance of data points from the mean. It is a robust alternative to standard deviation and is particularly useful when the data distribution is skewed.
3. Gini Coefficient:The Gini coefficient is a measure of inequality that can be applied to data variability. It ranges from 0 (perfect equality) to 1 (complete inequality).
The coefficient of variation is a versatile and powerful metric for assessing data variability in ML and intelligent signal processing applications. By providing insights into the relative dispersion of data points, CV aids in data normalization, feature selection, anomaly detection, signal denoising, and model evaluation. However, its limitations must be acknowledged, and robust statistical methods should be employed to minimize the impact of outliers. By carefully considering the data distribution and using appropriate best practices, practitioners can effectively harness the CV to gain valuable insights from data analysis and signal processing tasks.
4 out of 5
Language | : | English |
File size | : | 22688 KB |
Text-to-Speech | : | Enabled |
Enhanced typesetting | : | Enabled |
Print length | : | 148 pages |
Screen Reader | : | Supported |
Do you want to contribute by writing guest posts on this blog?
Please contact us and send us a resume of previous articles that you have written.
Novel
Page
Chapter
Genre
Library
E-book
Newspaper
Bookmark
Glossary
Preface
Synopsis
Scroll
Codex
Tome
Bestseller
Narrative
Autobiography
Memoir
Encyclopedia
Thesaurus
Narrator
Character
Catalog
Borrowing
Stacks
Archives
Research
Reserve
Reading Room
Rare Books
Special Collections
Interlibrary
Literacy
Study Group
Thesis
Dissertation
Storytelling
Awards
Reading List
Textbooks
Karuho Shiina
Joseph I Sirven
Rebecca Currington
Donna Danielle Mccartney
Mark Hallerberg
Christine Lynn Herman
Steve Chapman
Jo Avery
J E Murphy
Pravin Sawhney
Christoph Neuwirth
Hiromi Uehara
Ken Ludwig
Danni Quintos
Debamita Chakraborty
Paul Luebke
Mike Pinfold
Kevin Lewis
Darrin Doyle
Merita S Whatley
Light bulbAdvertise smarter! Our strategic ad space ensures maximum exposure. Reserve your spot today!
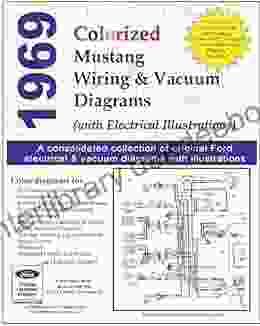

- George BellFollow ·6.8k
- Greg CoxFollow ·8.7k
- Christopher WoodsFollow ·19.7k
- Howard PowellFollow ·11.3k
- Jeffrey HayesFollow ·3.1k
- Leslie CarterFollow ·2.8k
- Holden BellFollow ·6.2k
- Darnell MitchellFollow ·4k
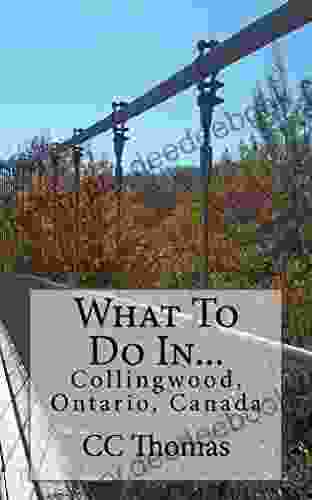

Discover the Enchanting Allure of Collingwood, Ontario,...
Nestled amidst the breathtaking landscape of...
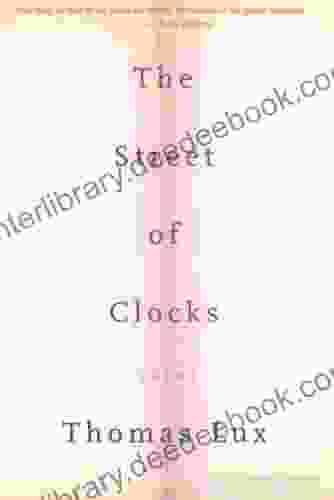

The Street of Clocks Poems: A Poetic Journey Through Time
Welcome to The Street...
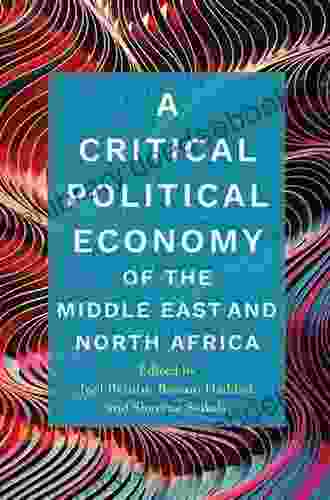

Critical Political Economy of the Middle East and North...
The Middle East and...
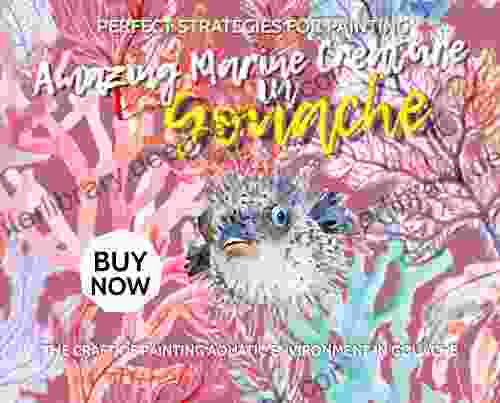

Perfect Strategies For Painting Amazing Marine Creatures...
Gouache is a...
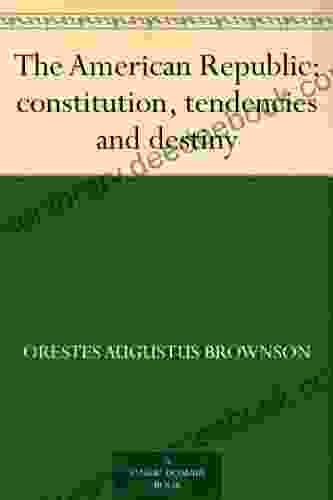

The American Republic: Constitution, Tendencies, and...
The American Republic,...
4 out of 5
Language | : | English |
File size | : | 22688 KB |
Text-to-Speech | : | Enabled |
Enhanced typesetting | : | Enabled |
Print length | : | 148 pages |
Screen Reader | : | Supported |